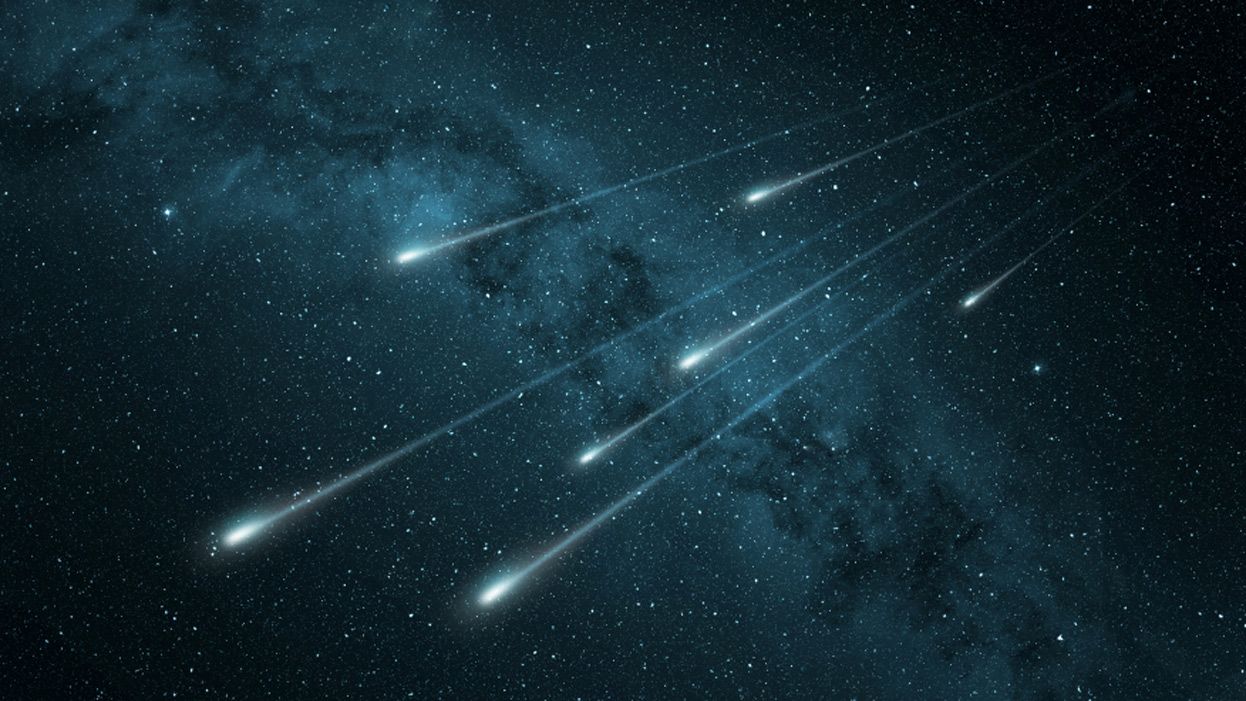
Bolides are bright fireballs (although not all fireballs are bolides), incredibly bright flashes of light emitted when a large meteoroid or asteroid enters Earth’s atmosphere and explodes. Until recently, there hasn’t been an effective way to identify and track bolides. Most enter Earth’s atmosphere over oceans, which means ground-based observatories don't detect them. Further, bolide events are rapid, maybe on 2/10s of a second. As a result, historically, only about a dozen or so bolide events have been detected per year.
Now, as part of NASA's Asteroid Threat Assessment Project (ATAP), SETI Institute data scientist Jeff Smith and his team, building on the work of researchers who conceived this approach, have developed a way to identify more bolides than was previously possible. Jeff and his team have built a machine learning-based bolide detection and light curve generation pipeline using the Geostationary Lightning Mapper (GLM). The GLM is onboard two GOES weather satellites and is used to track optical changes in Earth's atmosphere, indicating lighting. To date, this bolide detection pipeline has identified more than 2,600 bolides in just two years.
Currently, the GLM is onboard two satellites operated by NOAA, the National Oceanic and Atmospheric Administration, that support weather forecasting, severe storm tracking and meteorology research. The GLM captures momentary optical changes that indicate the presence of lightning, detecting millions of lightning strikes. The GLM is calibrated for lightning, not bolides. The task of manually sifting through the GLM data to find bolides is like searching for a needle in a haystack. The initial algorithms had a detection precision of about 1.5%, meaning 98.5% of the detections were not really bolides. By using supervised machine learning and more tightly defining filters, and manually labeling tens of thousands of bolide and lightning events throughout the training process to distinguish lightning from bolides, the new data pipeline has achieved precision approaching 80%. Thus, while it's still necessary for a human to review the lightcurve to confirm a positive bolide detection, the time required is substantially reduced.
For the most part, debris created during a bolide event burns up in Earth’s atmosphere. Any fragments that survive passage through the atmosphere and hit the ground is a meteorite. Most bolides detected are relatively small and do not pose a threat to life on Earth. However, even though it might be a once in a 50,000-year event, there’s the potential for a significant asteroid strike to do devastating damage. More than 66 million years ago, the asteroid that created the Chicxulub crater caused a mass extinction of dinosaurs. More recently, researchers have been studying the asteroid Apophis to assess its possible future risk to Earth. By detecting and tracking a significant number of bolides, it will be possible to develop models to predict where and how asteroids will enter Earth's atmosphere and assess the risk.
To learn more, you can read the research about this project published in Icarus here. Or, if you'd like to watch Jeff discuss his work during a recent SETI Live social media discussion, you can watch it here.