Frontier Development Lab
A Powerful Public-Private Partnership for Cutting-Edge AI/ML Research
The Frontier Development Lab (FDL) is a groundbreaking public-private collaboration between NASA, the US Department of Energy, and the SETI Institute, with the invaluable support of top AI/ML technology leaders and subject matter experts from the private sector. Notable partners include Google Cloud, NVIDIA, Lockheed Martin, Intel, the Luxembourg Space Agency, and Mayo Clinic.
With a remarkable eight years of operation, FDL is an exceptional platform, bringing together the brightest minds of early-career PhDs and postdoctoral researchers from diverse scientific backgrounds. These talents are paired with their counterparts in computer science, fostering a synergistic environment that drives this applied AI/ML research accelerator. FDL's primary goal is to tackle some of humanity's most critical research challenges.
FDL's mission is to solve pressing issues such as extreme weather modeling, disaster response and recovery, planetary defense, astronaut health, Heliophysics, Earth Science, astrobiology, and planetary science. Through this collaborative effort, FDL pioneers advancements that have the potential to revolutionize space and Earth science and contribute to the betterment of our world.
Learn more about FDL at http://fdl4ai.com
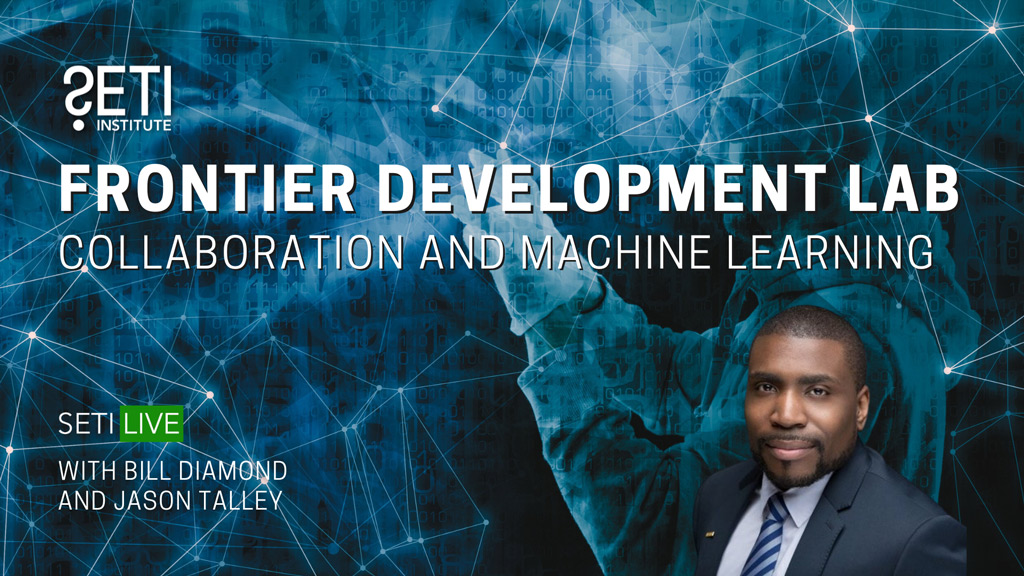
FRONTIER DEVELOPMENT LAB - Collaboration and Machine Learning
, 2:30 PM - 3:00 PM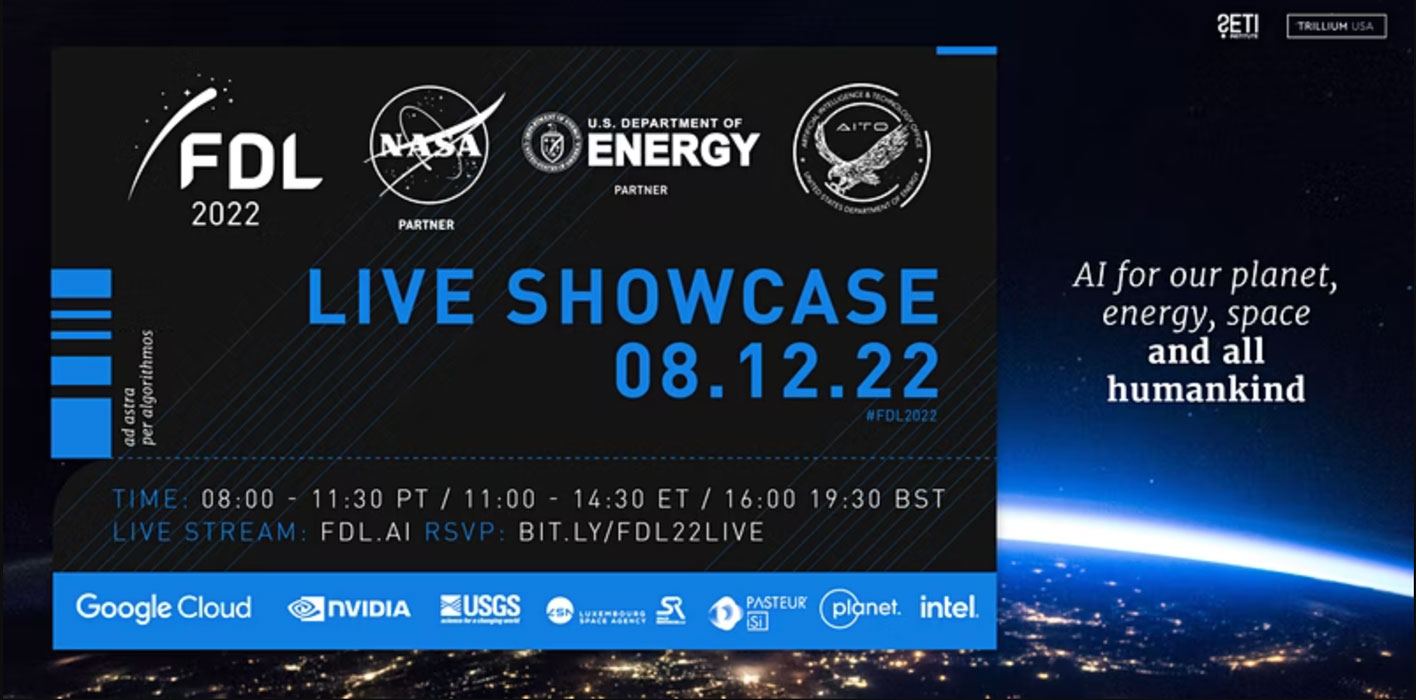
FDL 2022 Live Showcase Event
, 8:00 AM - 11:30 AM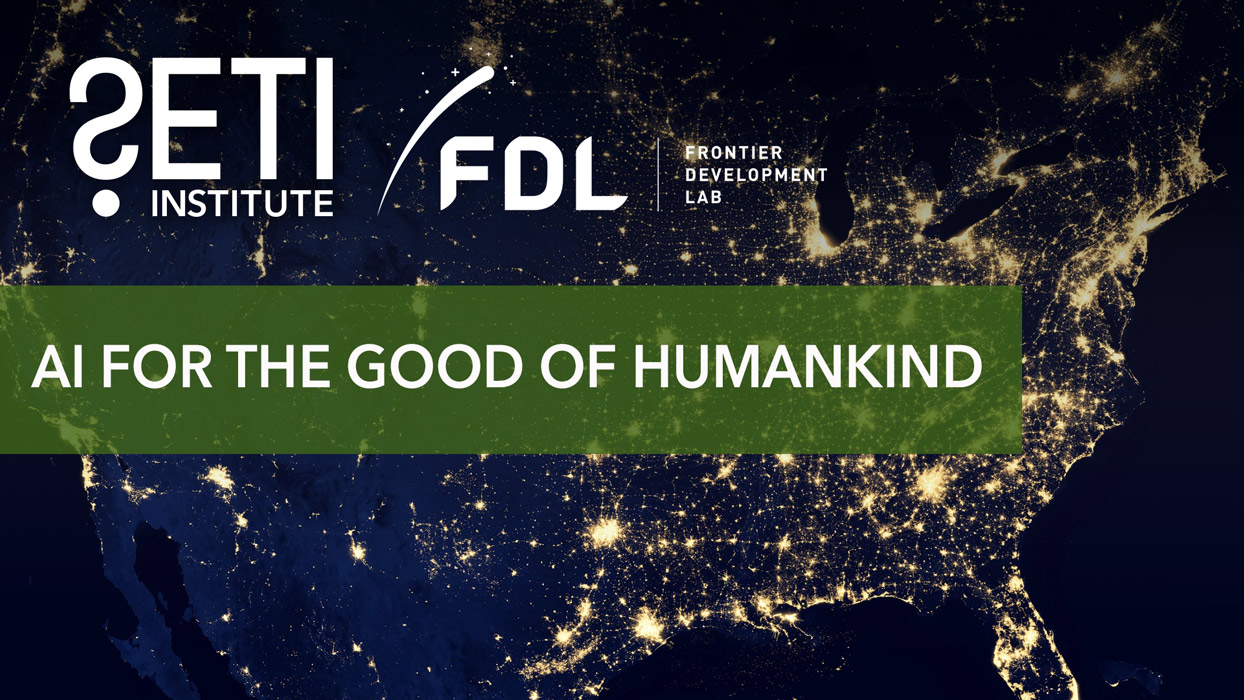
SETI Live - AI for the Good of Humankind
, 9:15 AM - 9:45 AM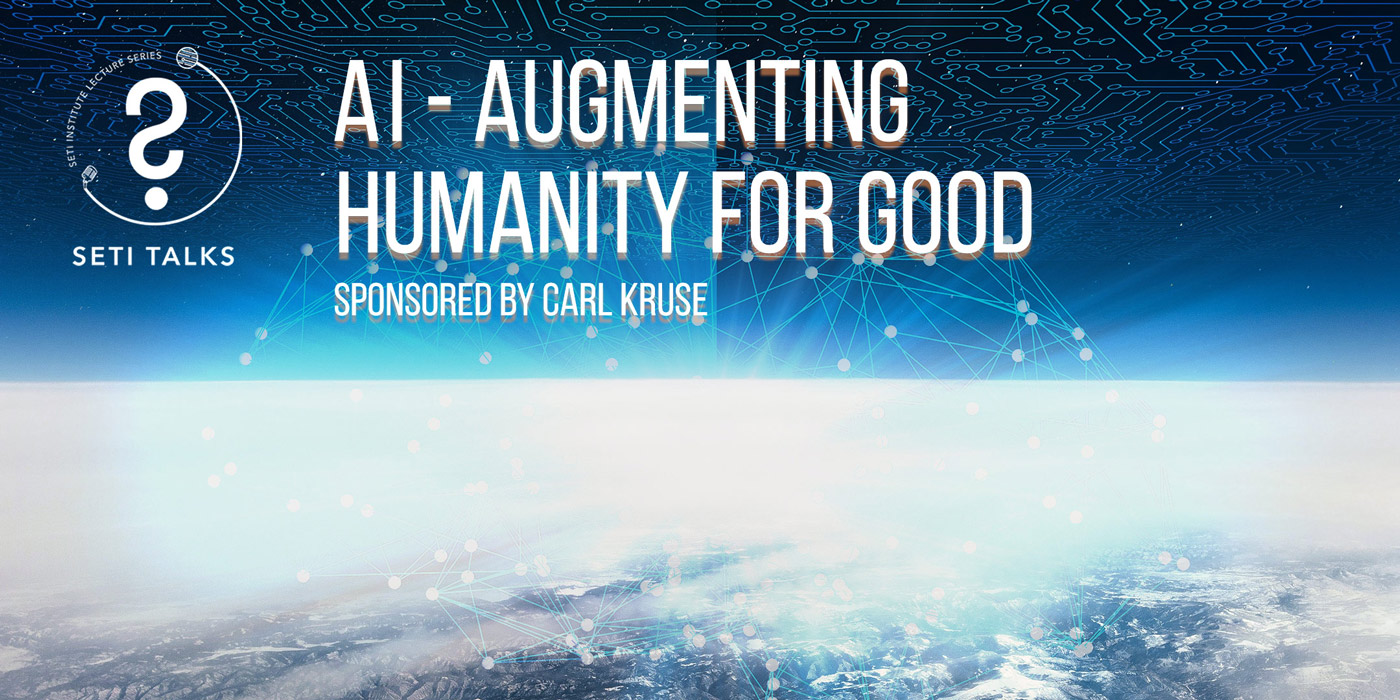