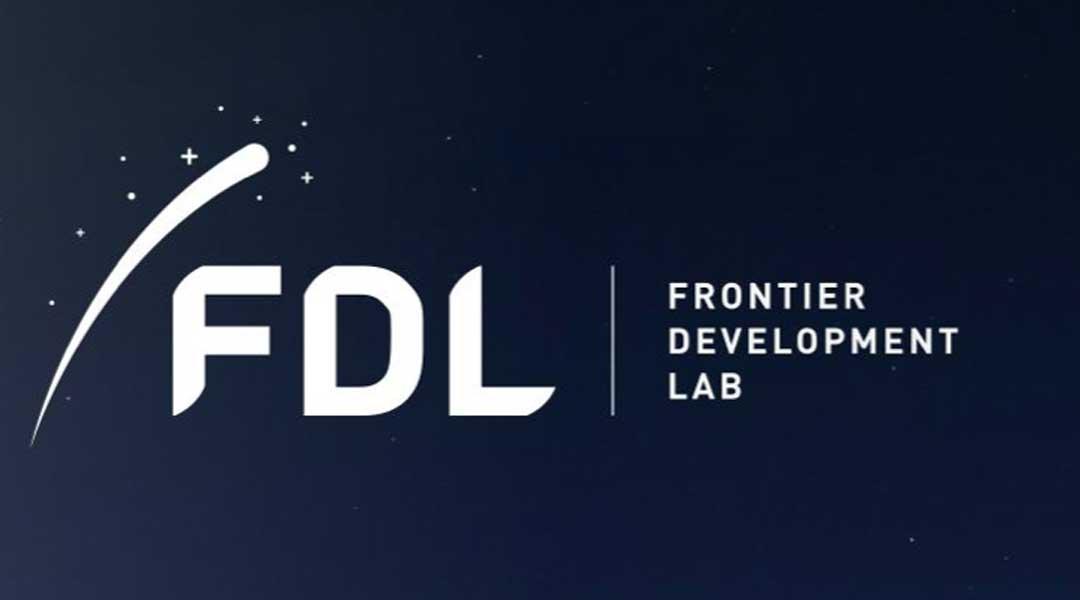
During the summer of 2020, Frontier Development Lab (FDL) continued to demonstrate Machine Learning's potential for scientific process and discovery and new paradigms of engineering, from predicting GPS scintillation to co-operative robots on the Moon.
Illustrative progress in science includes methods for predicting natural phenomena and the discovery of new physical insight to enable earlier decisions, better predictions and planning strategies within complex systems.
Artificial Intelligence is enabling an exciting new era of these capabilities. FDL's goal is to explore and apply these to problems critical to space science, exploration, and all humankind's benefit.
This year's Frontier Development Lab kicked off under highly unusual circumstances. Due to the COVID-19 pandemic, all eight US teams with NASA and the SETI Institute and three European teams with ESA and the University of Oxford worked in an entirely virtual environment. Once again, the teams achieved extraordinary results with novel approaches in applying AI for space science.
In addition to its primary scientific objectives, FDL 2020 was as much a social experiment for how high-performance teams can work together virtually and still deliver powerful and meaningful results. FDL is fundamentally about interdisciplinary teams applying AI and machine learning to challenges in research and exploration that are scalable, impactful and beneficial to all humankind. In the midst of a global pandemic, this year’s research questions were all the more challenging.
Eight US teams addressed questions in Earth science, planetary science, astronaut health and heliophysics (the science of the Sun and its physical connection to the solar system). In Europe, teams addressed questions on climate and digital twinning as well as space-traffic management.
The teams, comprised of early-career PhDs in AI/ML and multidisciplinary science domains, were supported by subject matter experts from NASA, including NASA Headquarters, NASA Ames Research Center and the NASA Marshall Space Flight Center. Google Cloud made the exceptional contribution of massive compute resources, without which FDL would not be possible. Additional support was provided by researchers at the SETI Institute, together with partners at the Mayo Clinic, USGS, Lockheed Martin, Luxembourg Space Agency, MIT Portugal, Planet Labs Inc, IBM and NVIDIA. FDL Europe was supported by ESA ESRIN and ESA ESOC and faculty and collaborators at the University of Oxford.
Partner organizations support the FDL program by providing funding, cloud compute, hardware, AI/ML algorithms and datasets, software, cloud storage resources, subject-matter experts and advisors.
US Teams
Starspot What can we learn about heliophysics from other stars? ML can learn from faint starlight observations to explain luminance dips through competing plausible hypotheses in terms of extrasolar transits, spot formation, and each star's rotational characteristics.
Geoeffectiveness How do the interactions of the solar wind affect our planet’s magnetosphere? ML can learn to reconstruct the causal pathways of solar wind interactions propagating through our ionosphere and aid scientists in piecing together space weather's effects on the Earth's magnetosphere.
Moon for Good 2 Can we see in perpetually dark Moon craters where water likely exists? ML can learn to enhance the light in faint images of permanently shadowed regions of craters, to aid sustainable missions on the Moon that depend on ice deposits.
Astronaut Health How does cancer evolve under high radiation environments, like Mars? ML can learn to isolate causes of cancer in high-dimensional heterogeneous omics data and help scientists design early interventions to reduce cancer risk during space missions.
Waters of the US Where are the Earth’s streams flowing right now, and how often? ML can learn to map water streams down to 5m wide and estimate their flow frequency daily by fusing high-resolution satellite imagery with LiDAR sensor data to create fundamentally new dynamic hydrology maps and help manage our water resources.
Lightning Can we improve forecasts of severe weather by looking for lightning from satellites? ML can learn to detect lightning and cloud plumes in a sequence of satellite images that indicate imminent severe weather and halve the false alarms produced by current systems.
Knowledge Discovery Framework Can we reverse image search on our EO archives? ML can learn to search for similar images in Earth Observation archives with decades of atmospheric imagery based on the characteristics of a single query image and help scientists curate our collective knowledge hidden in our archives
Earth Intelligence Engine How would future floods affect an area as seen from a satellite? ML can learn to generate synthetic satellite images of future coastal flooding that are physically consistent and photorealistic, enabling experts to communicate flood risks more effectively to decision-makers.
European Teams
Digital Twin Earth Can we lower the cost of accurate global precipitation forecasts? ML can learn to forecast precipitation by fusing simulated satellite weather data with physical model data to offer a low-cost alternative to expensive simulation infrastructure
Constellations Can we protect our satellites by predicting collisions with space debris? ML can learn to predict the timings of collision onsets from historical trajectory data while associating and explaining the collision risk through a set of key variables.
Clouds and aerosols How does pollution influence the formation of large marine clouds? ML can learn to track the motion of mesoscale clouds and find the causal influence of aerosol pollution on complex cloud behavior.
For full details, you can read the FDL 2020 Proceedings here.
About the Frontier Development Lab (FDL) Hosted in Silicon Valley by the SETI Institute, FDL is an applied artificial intelligence research accelerator developed in partnership with NASA's Ames Research Center. Founded in 2016, FDL aims to use AI technologies to challenges in scientific exploration by pairing machine learning expertise with science and exploration researchers from academia and industry. These interdisciplinary teams address tightly defined problems, and the format encourages rapid iteration and prototyping to create outputs with meaningful application to the space program and humanity.